Join our daily and weekly newsletters for the latest updates and exclusive content on industry-leading AI coverage. Learn More
H2O.ai, a provider of open-source AI platforms, announced today two new vision-language models designed to improve document analysis and optical character recognition (OCR) tasks.
The models, named H2OVL Mississippi-2B and H2OVL-Mississippi-0.8B, show competitive performance against much larger models from major tech companies, potentially offering a more efficient solution for businesses dealing with document-heavy workflows.
David vs. Goliath: How H2O.ai’s tiny models are outsmarting tech giants
The H2OVL Mississippi-0.8B model, with only 800 million parameters, surpassed all other models, including those with billions more parameters, on the OCRBench Text Recognition task. Meanwhile, the 2-billion parameter H2OVL Mississippi-2B model demonstrated strong general performance across a range of vision-language benchmarks.
“We’ve designed H2OVL Mississippi models to be a high-performance yet cost-effective solution, bringing AI-powered OCR, visual understanding, and Document AI to businesses,” Sri Ambati, CEO and Founder of H2O.ai said in an exclusive interview with VentureBeat. “By combining advanced multimodal AI with efficiency, H2OVL Mississippi delivers precise, scalable Document AI solutions across a range of industries.”
The release of these models marks a significant step in H2O.ai’s strategy to make AI technology more accessible. By making the models freely available on Hugging Face, a popular platform for sharing machine learning models, H2O.ai is allowing developers and businesses to modify and adapt the models for specific document AI needs.
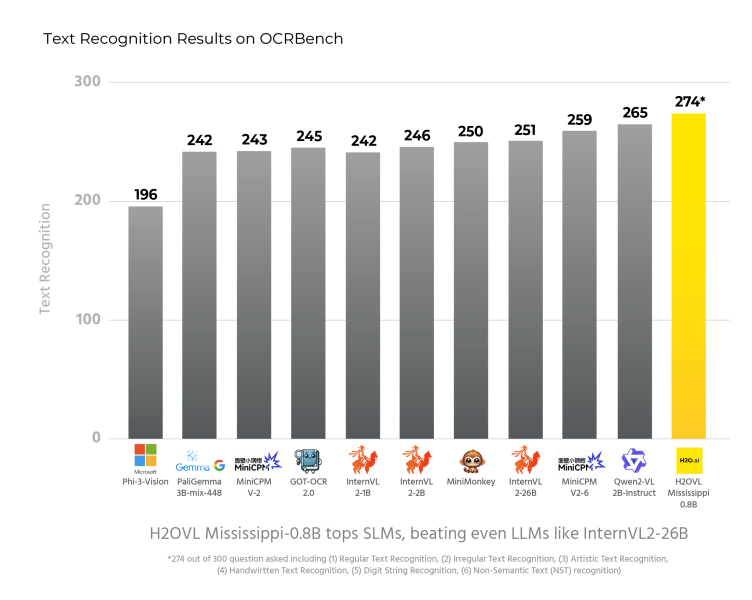
Efficiency meets effectiveness: A new approach to document processing
Ambati highlighted the economic advantages of smaller, specialized models. “Our approach to generative pre-trained transformers stems from our deep investment in Document AI, where we collaborate with customers to extract meaning from enterprise documents,” he said. “These models can run anywhere, on a small footprint, efficiently and sustainably, allowing fine-tuning on domain-specific images and documents at a fraction of the cost.”
The announcement comes as businesses seek more efficient ways to process and extract information from large volumes of documents. Traditional OCR and document analysis methods often struggle with poor-quality scans, challenging handwriting, or heavily modified documents. H2O.ai’s new models aim to address these issues while offering a more resource-efficient alternative to larger language models that may be excessive for specific document-related tasks.
Industry analysts note that H2O.ai’s approach could disrupt the current landscape dominated by tech giants. By focusing on smaller, more specialized models, H2O.ai may be able to capture a significant portion of the enterprise market that values efficiency and cost-effectiveness.
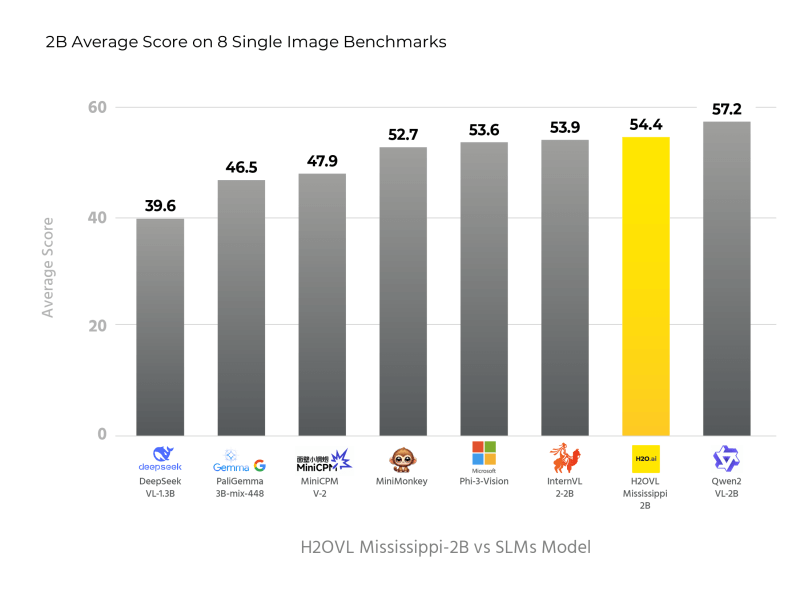
Open source and enterprise-ready: H2O.ai’s strategy for AI adoption
“At H2O.ai, making AI accessible isn’t just an idea. It’s a movement,” Ambati told VentureBeat. “By releasing a series of small foundational models that can be easily fine-tuned to specific tasks, we are expanding the possibilities for creating and using AI.”
H2O.ai has raised $256 million from investors including Commonwealth Bank, Nvidia, Goldman Sachs, and Wells Fargo. The company’s open-source approach and focus on practical, enterprise-ready AI solutions have helped it build a community of over 20,000 organizations and more than half of the Fortune 500 companies as customers.
As businesses continue to grapple with digital transformation and the need to extract value from unstructured data, H2O.ai’s new vision-language models could provide a compelling option for those looking to implement document AI solutions without the computational overhead of larger models. The true test will be in real-world applications, but H2O.ai’s demonstration of competitive performance with much smaller models suggests a promising direction for the future of enterprise AI.